
There is growing interest in systems that generate timeline summaries by filtering high-volume streams of documents to retain only those that are relevant to a particular event or topic. Continued advances in algorithms and techniques for this task depend on standardized and reproducible evaluation methodologies for comparing systems. However, timeline summary evaluation is still in its infancy, with competing methodologies currently being explored in international evaluation forums such as TREC. One area of active exploration is
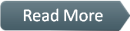